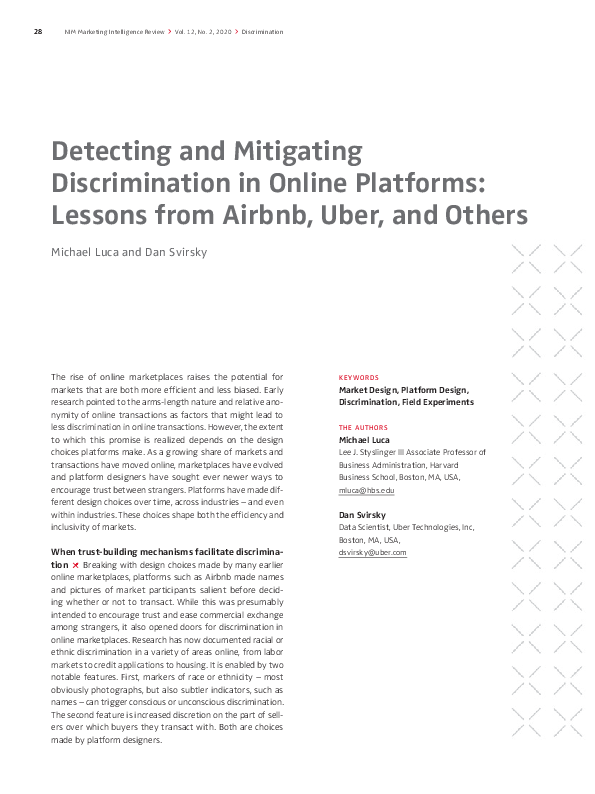
Detecting and Mitigating Discrimination in Online Platforms: Lessons from Airbnb, Uber, and Others
Michael Luca and Dan Svirsky
Research has documented racial or ethnic discrimination in online marketplaces, from labor markets to credit applications to housing. Platforms should therefore investigate how platform design decisions and algorithms can influence the extent of discrimination in a marketplace. By increasing awareness of this issue, managers can proactively address the problem. In many cases, a simple but effective change a platform can make is to withhold potentially sensitive user information, such as race and gender, until after a transaction has been agreed to. Further, platforms can use principles from choice architecture to reduce discrimination. For example, people have a tendency to use whatever option is set as the default. If Airbnb switched, for instance, its default to instant book, requiring hosts to actively opt out of it, the company could reduce the scope for discrimination. It is important that discrimination and possible solutions are discussed transparently.